Query Plan Regression Testing in GraphQL Federation
TL;DR:
We built a tool that exports all Schemas and GraphQL Operations from all our customers into a private GitHub repo. When we change the Cosmo Router, we run the Router in "headless Query Planning mode" against the exported queries and check if the query plans are the same as the previous version. This ensures that we never accidentally introduce a breaking change to our customers.
Cosmo Router serves billions of requests every day. If a Query cannot be planned (anymore), a customer-facing query will fail, resulting in a broken experience for the end user. Our goal is to do everything in our power to avoid that.
In this post, I want to share the story of a tool I recently built to solve this problem. It's not a flashy tool, and it's not even complicated, but it solves a very specific—and very real—problem.
The tool has three main parts:
- A Router cmd that generates query plans for a set of GraphQL Operations in a given directory.
- A daily GitHub workflow that exports all Schemas and GraphQL Operations from our customers to a private GitHub repo
- Another GitHub workflow that runs every time a change is introduced to the Cosmo Router and alerts us if query plans change or new errors appear.
Shipping this tool is part of our ongoing effort to improve the quality of the Cosmo Router. We've got big plans for new features in GraphQL Federation, so it's our top priority to ensure we can iterate fast and ship confidently.
The Problem: The Query Planner is never 100% done
Query Planning in GraphQL Federation is a complex problem, especially with the introduction of v2
, which introduced many new directives and features that can lead to very complex query plans.
One of the biggest challenges in Federation Query Planning is the lack of a specification for how the query planner should work, and the fact that Federation is less strict than it could be. In practice, we can borrow an idea from Hyrum's Law .
Here's the gist of it:
With a sufficient number of users of an API, it does not matter what you promise in the contract: all observable behaviors of the system will be depended on by somebody.
Consequently, the current "Specification" for the Query Planner is effectively the sum of all behaviors observed in the wild. By capturing all observed behaviors—meaning we persist all Query Plans across all customers—we get as close as possible to having a specification, without formally defining one.
So, that's what we do.
But before we dive into the solution, it's important to know what Query Plans are and why they matter.
Query Plans in GraphQL Federation: What They Are and Why They Matter
One of the main jobs of the Cosmo Router is figuring out how to fetch the data for a query—what subgraphs to call, in what order, and with what arguments. That set of decisions is what we call a Query Plan.
Here's a simple example of a Query Plan:
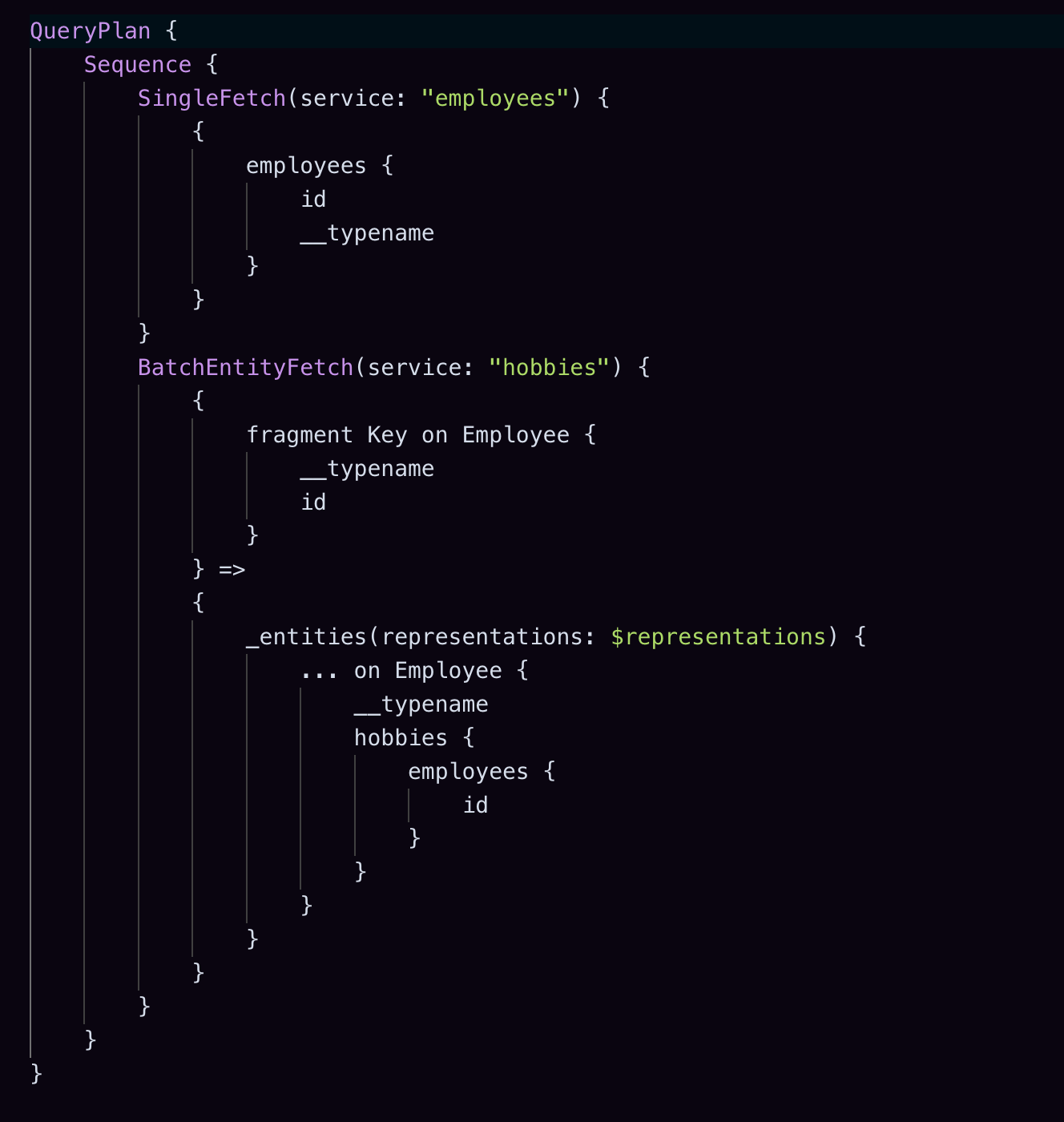
Query Plans serve different purposes for different stakeholders. For a frontend developer, the Query Plan can be used to understand how the query will be executed. In case of a performance issue, they could take a look at the Query Plan to get an idea of which parts of the query are slow. In addition, the Query Plan can tell them which Subgraphs their Query depends on. This could be useful for them to understand who to talk to if they have a question about performance or a feature request.
For a backend engineer or anyone responsible for implementing a subgraph, Query Plans give helpful information about Query Patterns. You can look at the Supergraph schema and understand which part your Subgraph is responsible for, but that doesn't tell you how it is being used to resolve commonly occurring queries. By looking at different Query Plans, a backend engineer can understand hotspots in the graph and potential areas for improvement. For example, they could work with other Subgraph owners to see if they can move a field to a different Subgraph to eliminate a bottleneck.
Platform engineers are another stakeholder that benefits from Query Plans. They're responsible for the overall performance of the federated graph, and Query Plans allow them to find problems in the structure of the graph. By looking at Query Plans from a high-level perspective, they can spot patterns causing performance issues. More specifically, the graph may be divided in a way that causes unnecessary "jumps" between Subgraphs. In such cases, teams can work with Subgraph owners to reassign field ownership and reduce the number of jumps.
For the Router, the Query Plan is a set of instructions on how to load the data to be able to resolve a query. It's typically a set of fetches grouped into sequential and parallel steps. Part of the query planning process involves figuring out which fetches can be executed in parallel and which must occur sequentially. In addition, the Query Plan also contains information on how to merge the data from the fetches, and how to render the final result.
Side Trek: A Deep Dive into Query Planning Performance
Want to dive deeper into how query planning affects performance? Check out Jens' GraphQL Summit talk about how Dataloader 3.0's approach dramatically improved query execution:
Now that you've had a chance to learn about Query Planning's impact on performance, let's look at how we have built tools to catch changes.
Router Subcommand for GraphQL: Making Query Plans Easy to Diff
The first step in solving the problem was giving ourselves—and our customers—an easy way to actually see the query plan changes. So, I took some of the code already made by a colleague (thanks, Sergiy!) and built a new subcommand for the Cosmo Router that outputs Query Plans in a format that's easy to compare.
With this, you can generate and diff all your Query Plans against a previous version. It's a simple way to see what changed and where. For example, maybe a fetch was removed, or a new field caused a plan to split differently across services. Before this tool existed, there wasn't an easy way to spot those kinds of differences.
We originally built this with enterprise customers in mind. They need a way to run these checks before they deploy changes to their subgraphs, and in large systems where even a tiny change can trigger unexpected side effects, this is especially important.
Now, with this subcommand, anyone can supply a folder of queries and generate their plans locally. All you need is the queries you want to check and the router version you're testing against; no special setup is required.
CI Integration for GraphQL Query Planning with GitHub Actions
The Router subcommand gave us visibility, but we needed something more proactive. So, we added a GitHub workflow that runs automatically on every pull request (PR).
Here's how it works: When someone opens a PR that touches the Router, CLI, or engine, the workflow starts. It downloads the exact versions from the PR and runs the Query Planner against a set of actual customer queries (collected via metrics, only from customers who've opted in). The workflow blocks the merge if something breaks or a query plan changes unexpectedly.
This check doesn't just catch runtime errors. It will flag subtle changes in the shape of the plan so engineers can review them and make sure they're intentional. Sometimes a plan changes for a good reason—like introducing a new feature—but we want to know that, not be surprised by it later.
Below is what it looks like when a query plan fails or changes unexpectedly (uuid is scrubbed for privacy):
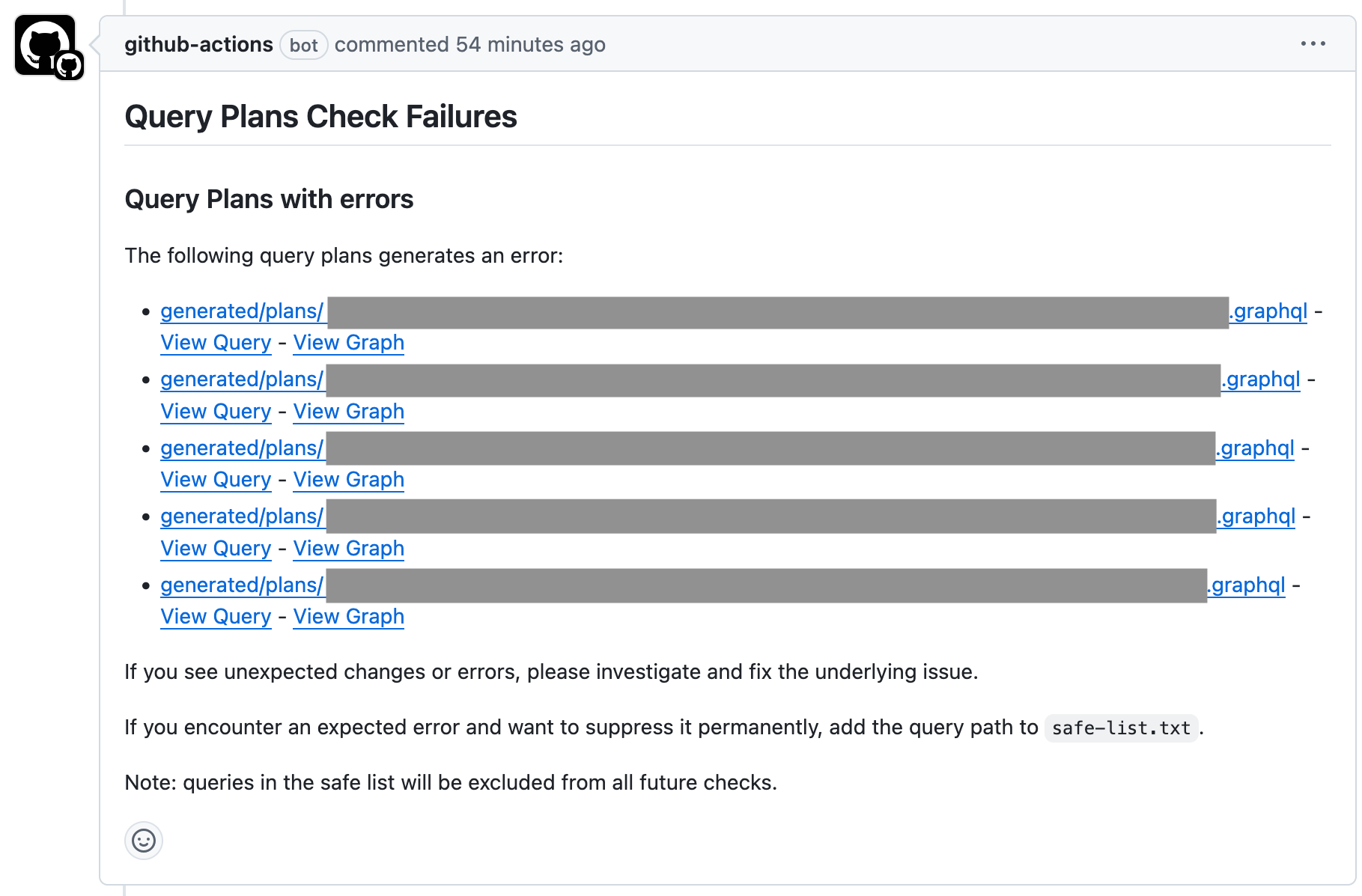
And this is what it looks like when everything passes cleanly:
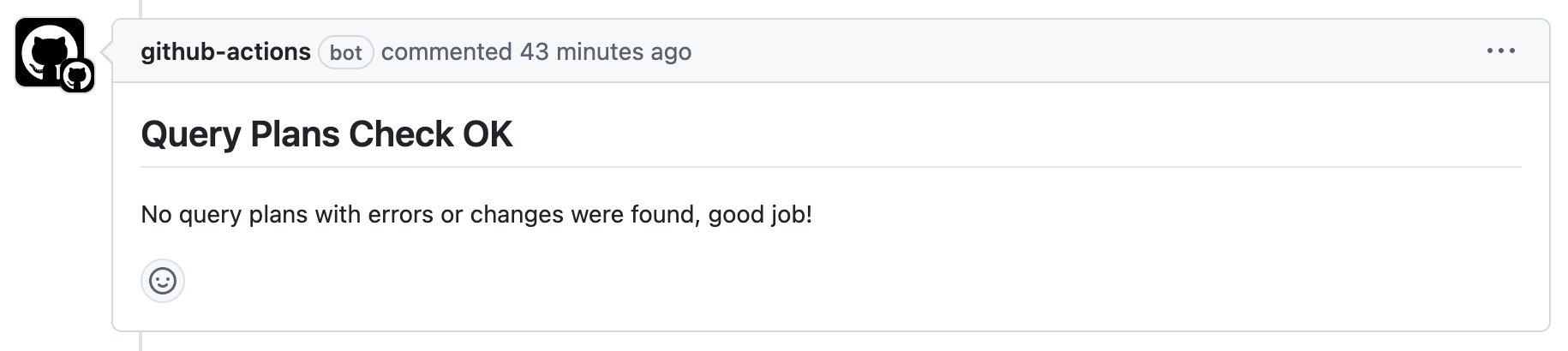
Each PR runs in isolation, so we don't run into merge conflicts or stale data. It is wired directly into our CI. This setup gives us confidence that changes won't introduce regressions—without needing to test every customer setup manually. In addition, we can review Query Plan changes when we intentionally introduce them, ensuring that they match our customers' expectations.
So how does this all work behind the scenes?
Under the Hood: Snapshotter + Query Planner Tester
Two main components make this work behind the scenes: the snapshotter and the Query Planner Tester.
The snapshotter is a service that regularly pulls in the operations and the execution config our customers are running in production (again, only if they've opted in via metrics). It doesn't include any data, just the queries themselves and the execution config. These are saved as snapshots and organized by tenant.
Then there's the Query Planner Tester—a GitHub Action that runs those snapshots against the specified Router and CLI versions. It generates the query plans and checks for any changes or errors. If something looks off, the PR will be blocked until a human can review it.
And because each workflow runs on its own branch, it can scale cleanly, even when multiple PRs are open at the same time. Of course, running these checks at scale comes with its own challenges.
Performance Notes: CI-Friendly, CPU-Hungry
Query Planning is unsurprisingly CPU-intensive. It doesn't use much memory, but it does push the processor. Since we're running these checks inside GitHub-hosted CI workflows, we have to keep an eye on how long things take—especially now that we're checking queries from every opted-in customer on every PR.
So far, the runtimes have been reasonable, but we're still monitoring closely. If it starts to slow things down too much, we have options: Optimize the planner logic or scale up to larger GitHub runners. Either way, it's better for it to take a bit longer and catch a breaking change than to merge something that breaks production.
Keeping federation velocity and query plan stability in balance is tricky. Like juggling on a unicycle, it takes coordination, timing, and a steady hand.
That’s why this tooling matters.
Conclusion: Quality as a Culture
This kind of tooling isn't glamorous. It doesn't ship new features or show up in a product demo. But it's the kind of quiet, behind-the-scenes work that is designed to keep everything else running smoothly.
You can't rely on best intentions alone when operating at scale—with hundreds of subgraphs, thousands of queries, and multiple teams contributing. You need guardrails. You need automation. You need confidence.
This team effort reflects something we care deeply about: building with care, thinking ahead, and never settling. Because in the never-ending quest for quality, every little step counts.
It's also a reminder that when there's no official spec to lean on, the only real guide is how things behave in the wild—and how we choose to respond to that.
Which leads to an interesting question:
If Hyrum's Law is true, is a very comprehensive test suite better than a specification?